MCA 2017: Computational Inverse Problems: from Multiscale Modeling to Uncertainty Quantification Symposium
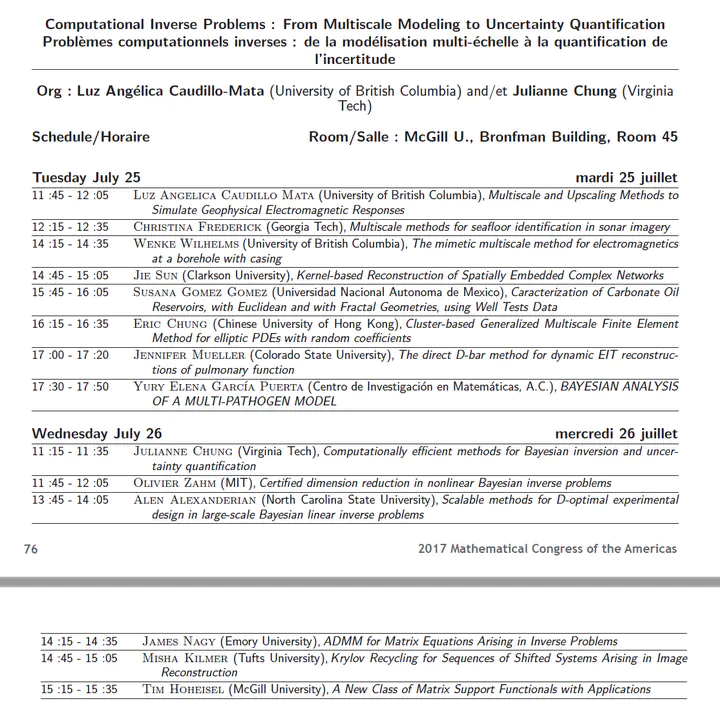
Special session (minysimposium) held as part of the Mathematical Congress of the Americas 2017.
Roles:
- Co-organizer
- Speaker
Period:
- July, 2016 to August 2017
Abstract:
Computational inverse problems are prevalent and essential in various scientific and industrial
applications, ranging from biomedical and geophysical imaging to computer vision and
neuroscience. For example, in many physical systems, measurements are obtained on the
exterior of an object (e.g., the human body or the earth’s crust), and they are used with the goal of estimating the internal structure(s) or physical properties of such object.
Currently, the main challenges in the field of inverse problems are (1) the affordable computation of the forward model, especially for models with multiple length-scale features, (2) the efficient and reliable computation of solutions to inverse problems, and (3) the development of statistical methods to validate model assumptions and inversion estimates. Sophisticated tools from statistics, numerical optimization, numerical linear algebra, and multiscale analysis are required to address these challenges.
The goal of this special session is to gather a diverse and accomplished pool of experts working in the active and thriving research area of computational inverse problems who will present an overview of the state of-the-art methods to address the key challenges discussed. For example, speakers will present on new nonlinear optimization approaches, novel regularization techniques, iterative reconstruction techniques (e.g., hybrid methods and preconditioners), methods for statistical validation and uncertainty quantification, and the progress on multiscale methods and their applications within an inversion procedure.